Tenyx Announces rsLoRA, An Advance in Efficient Fine-Tuning of LLMs
%20(1).png)
Tenyx Announces rsLoRA, An Advance in Efficient Fine-Tuning of LLMs
Tenyx has released a new parameter efficient method for fine tuning Large Lanuage Models (LLMS). As its name implies, “Rank-Stabilized LoRA (rsLoRA)" (or rsLoRA) improves on the popular LoRA (Low Rank Adaptation) method.The method has been recently featured on Hugging Face’s community blog posts.
LoRA uses auxilliary matrices (called "adapters") of varying rank (roughly, low rank = low complexity) to reduce the number of parameters to be modified during fine-tuning. One of the important findings of the original LoRA work was that fine-tuning with very low adapter rank (i.e. 4 to 32) could perform well, and that this performance did not improve further with increasing rank.
However, it turns out that this saturation of performance with very low rank is not primarily due to a very low intrinsic dimensionality of the learning manifold, but rather, it stems from the stunted learning of LoRA outside of very low adapter ranks.
Tenyx's rsLoRA rseearch demonstrates, both theoretically and empirically, this limitation of LoRA, and corrects for it with the rsLoRA method. To find out more, you can view the full blog here, and paper about the method here.
Browse more
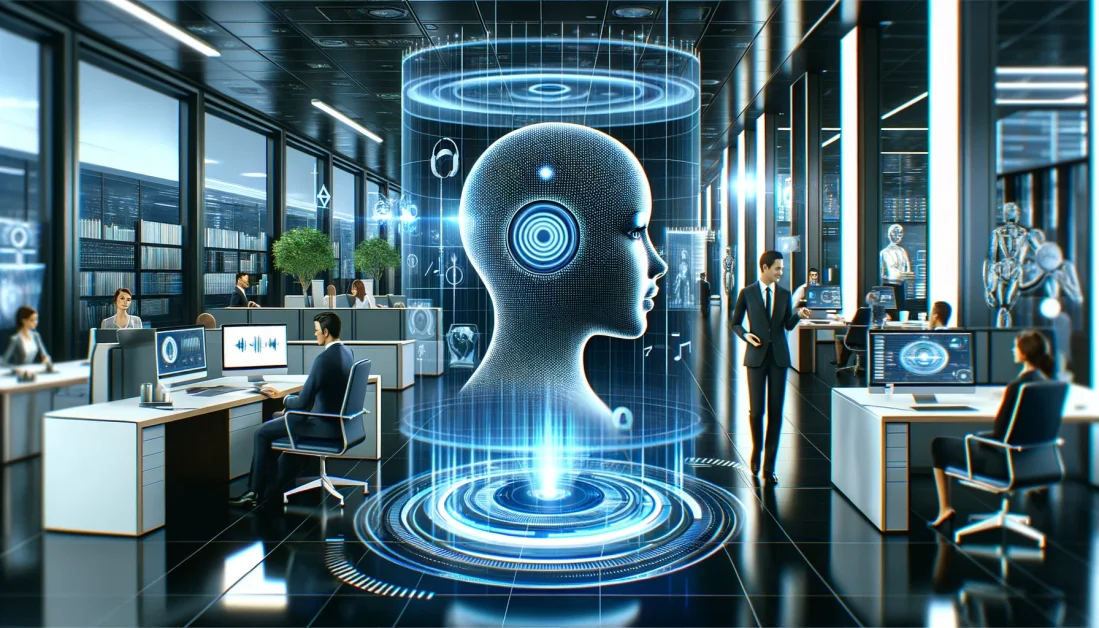
AI-Powered Voice-based Agents for Enterprises: Two Key Challenges

Tenyx introduces new conversational AI voice solution to elevate enterprise customer support
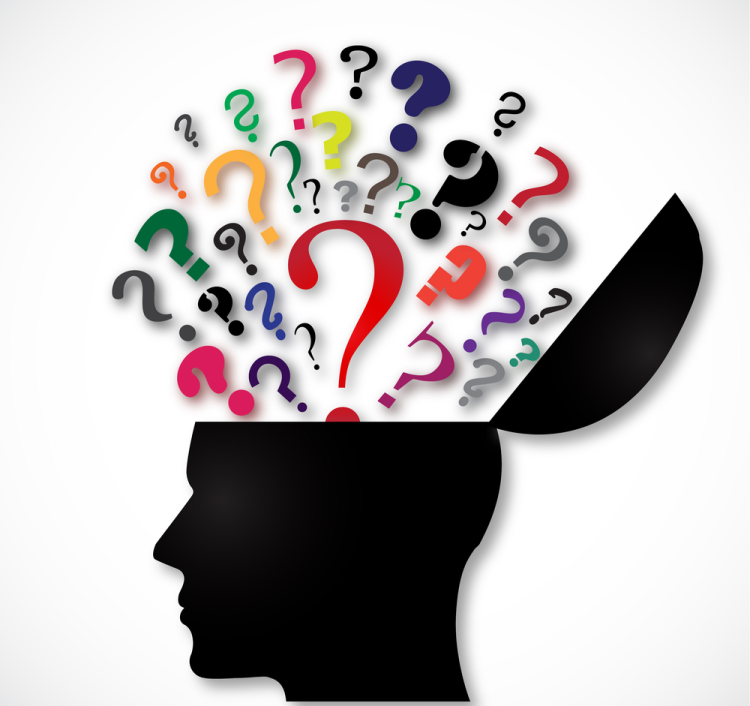